
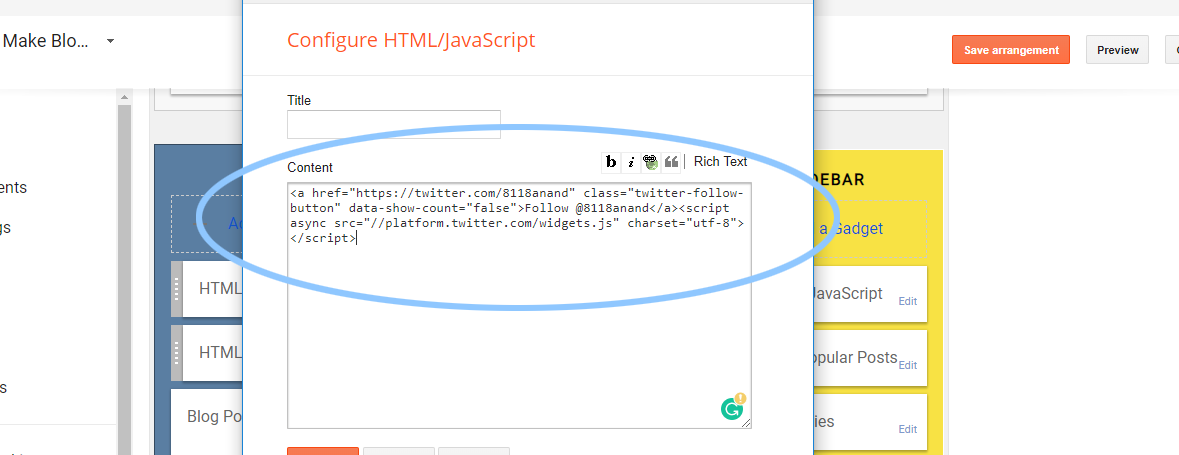
Dependency parsers, like the Stanford Parser, doesn't handle ungrammatical text very well because they were trained on corpuses like the Wall Street Journal. Building an accurate parser for tweets.Here's an example: "Just chomped my way through a massive apple, was pretty tasty. It would be good to be able to segment these two different ideas out. Determine context switches. Sometimes tweets contain two different ideas.Target-dependent Twitter Sentiment Classification.not about the term : "You should Google that.".The "aboutness" problem. Given a tweet, automatically detect if the sentiment is towards an entity.For example, in the phrase "Stanford is better than Berkeley", the tweet would be considered positive for both Stanford and Berkeley using our bag of words model because it doesn't take into account the relation towards "better". Our bag of words model doesn't handle comparisons very well.
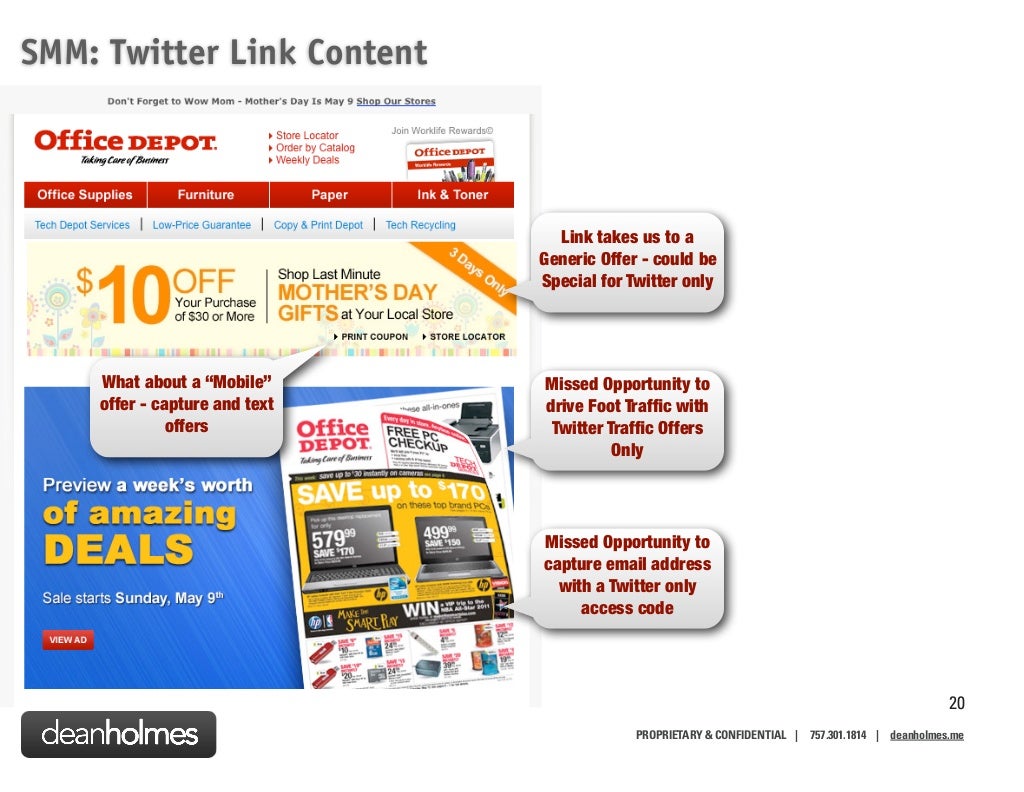
What's great and what's not: learning to classify the scope of negation for improved sentiment analysis. Councill, Ryan McDonald, and Leonid Velikovich. Words like no, not, and never are difficult to handle properly.
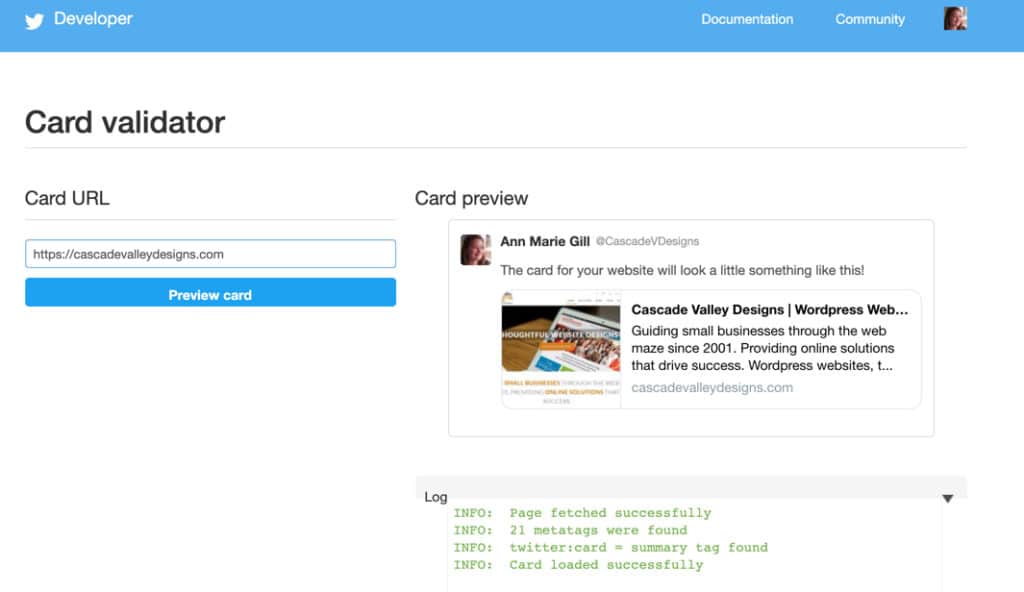
We haven't looked at classifying tweets with sentiment vs. objective tweets. We've focused mostly on classifying positive vs.
